People
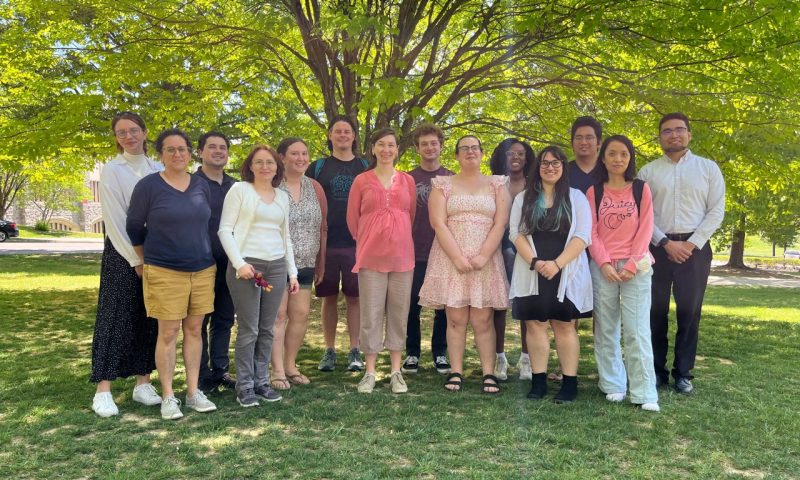
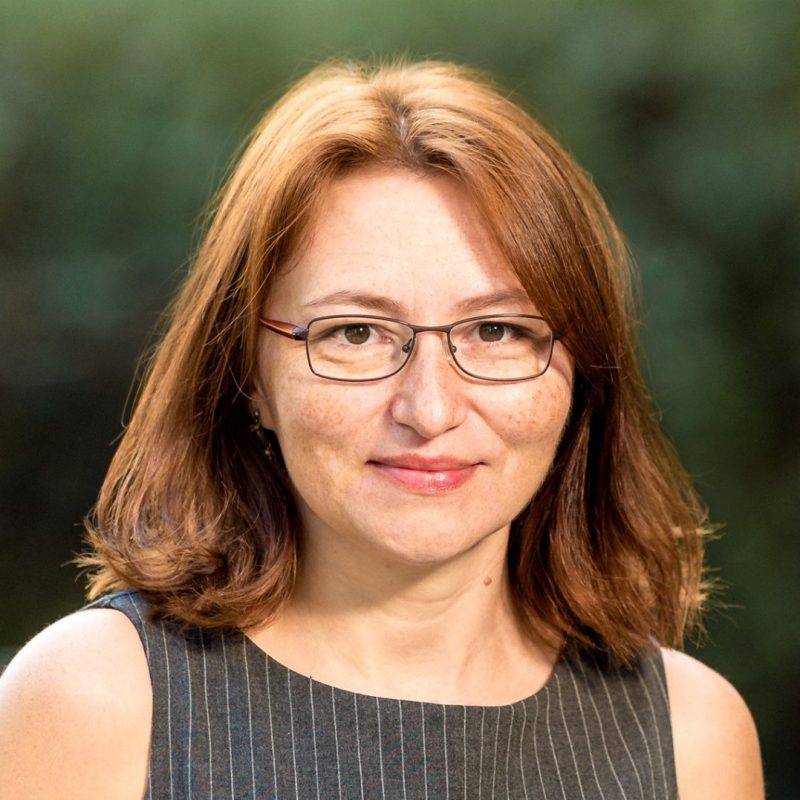
Virus dynamics; theoretical immunology; multiscale models of infectious diseases.

My research is based in dynamical systems and control theory, with a focus on multi-agent systems and their collective behavior. In this context, I am interested in biologically-inspired mathematical modeling, studying models from both analytical and numerical perspectives, and developing applications informed by the results. My work is inherently interdisciplinary and thus in line with the goals of VT-CMB. In addition, we use quantitative methods to understand how the behavior of individuals drives group-level complexity. Insights from my work in this area currently will shed light on how stress travels in human crowds, which has the potential to positively impact human condition in the future.
Wearing a blue pullover adorned with an orange-colored necklace, Assistant Professor Lauren Childs poses near Torgersen Hall.

My research program involves the development, analysis, and simulation of mathematical and computational models to examine biologically-motivated questions. A main focus of my work involves modeling with diverse quantitative techniques for a wide variety of applications in epidemiology, immunology, ecology and their interfaces. The construction and analysis of the models utilizes mathematics primarily from differential equations, dynamical systems and stochastic analysis. My work is highly interdisciplinary with collaborations in a variety of applied fields (e.g., biology, biochemistry, ecology, engineering, epidemiology, public health) at Virginia Tech and beyond.

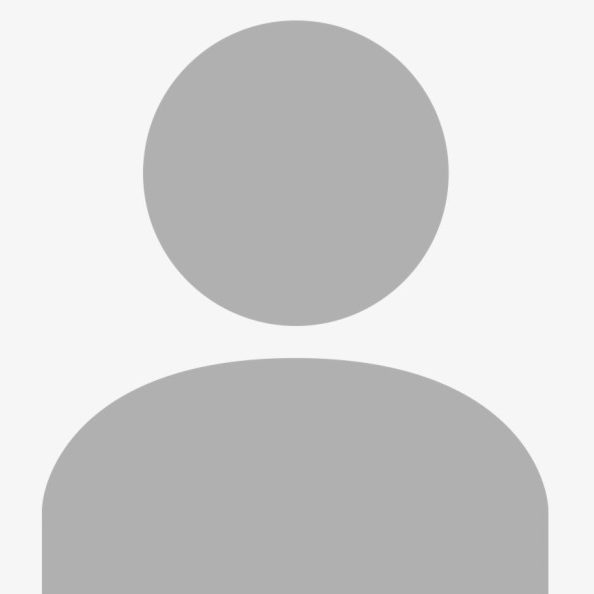

The Brown Lab utilizes theoretical methods, including molecular modeling and simulation, to gain mechanistic insight into disease pathways at an atomistic level. Our overarching goal is twofold: to employ these techniques to answer fundamental biological questions and to advance the methodology and techniques for understanding events such as protein aggregation and protein-membrane interactions.
We actively collaborate on multiple computer-aided drug discovery projects, employing a combination of free energy calculations, pharmacophore modeling, and virtual screening, to improve ranking accuracy and small molecule prediction power. Our focus is on generating new compound class leads and guiding the development of derivatives for prospective drug candidates targeting a wide range of biological targets and disease states.

The Chen Lab builds theoretical and computational models to investigate biological dynamics at the molecular, cellular and cell population levels, and to understand how they mediate biological functions. Cells are highly dynamic: gazillions of dynamic processes are happening in a cell at any moment in order for the cell to sense, respond and adapt to their environments, to move around, to develop and reproduce, to evolve, and even to maintain homeostasis. Experimental data often focus on one or few snapshots or aspects of these complex dynamics, and cannot provide a coherent mechanistic picture about how they work. We build mathematical models based on carefully crafted assumptions derived from experimental data, horizontal comparison, and established physical and chemical principles. Our modeling works aim to fill the gaps in the data, offer mechanistic insights, generate testable hypotheses, and aid future experimental design. To build models that are relevant and useful, we work in close collaboration with experimental groups. Our current research topics are largely concentrated in three broad areas: mechanics and signaling in mitosis, mechanics and control of bacterial motility, dynamics and regulation of gene expression.

Research in the Cimini lab focuses on how errors during mitotic cell division lead to abnormal chromosome numbers in the cell progeny and how abnormal chromosome numbers affect cell phenotypes and behaviors. Importantly, abnormal chromosome numbers are ubiquitously observed in cancer cells, but our understanding of the causes and consequences of such abnormalities is still limited. To achieve a mechanistic understating of events that ensure accurate chromosome segregation during mitosis and to investigate how cell populations evolve after abnormal cell division, my lab often collaborates with mathematical modelers. Through such collaborations, we have developed biophysical models that explain chromosome movement in mitotic cells (Civelekoglu-Scholey, He, et al., J Cell Biol, 2013), 3-D models of spindle assembly (Paul et al., PNAS, 2009; Silkworth et al., Mol Biol Cell, 2012), and population dynamics models to study the behavior and/or evolution of cell populations with abnormal chromosome numbers (Rutledge et al., Sci Rep, 2016; Baudoin et al., eLife, 2020). Our models have helped us understand our experimental observations and generate new hypotheses and predictions, which we then test by designing new experiments.
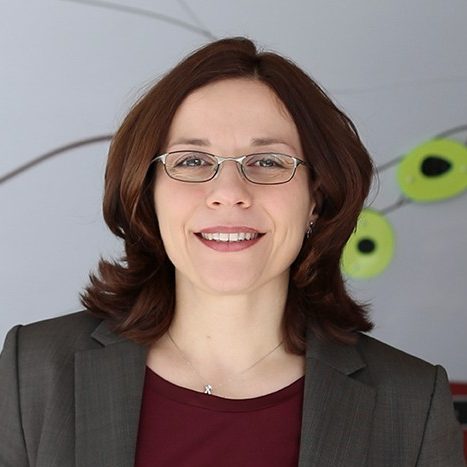
Research in my lab focuses on characterizing the mechanical properties of biological systems ranging from cellular components to tissues, with special emphasis on the development of new mathematical models and experimental methods. Although my research interests are diverse and continuously evolve over time, the common thread that runs through much of my work is my genuine passion in advancing fundamental and mechanistic knowledge of biological systems. This knowledge is crucial for the development of effective interventions to prevent and treat illness and disability.
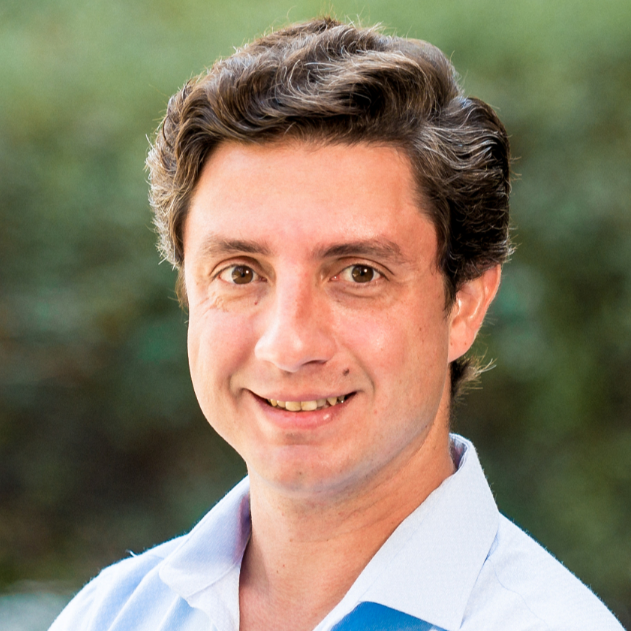
My group develops mathematical theories and simulations for the mechanobiology of complex sensing and shape change of animal cells and the dynamics bioinspired synthetic systems (chemically responsive hydrogels, biomineralization-inspired growth and form of composites through chemical precipitation). The three overarching themes of my research are mechanics, geometry, and reaction-transport phenomena. To this end, my research employs the following tools: solid mechanics, fluid mechanics, differential geometry, finite element analysis, and more recently deep learning. I often collaborate with experimentalists to devise general theoretical frameworks motivated by experiments. I also have ongoing projects focused on computational algorithm development: (i) My group is developing a variational physics-informed neural network algorithm to directly and efficiently minimize a functional (i.e., Lagrangian, Hamiltonian) to find the physical states of a system in lieu of solving the corresponding equations of motion, which can be computationally costly to determine all possible solutions. (ii) We are developing a versatile shape recognition algorithm by analyzing the curve geometries that bound a shape by employing the geometric invariants of space curves. The projects (i) and (ii) have important implications, respectively, for tackling complex problems in biology that can be represented by differential equations, and for shape recognition, classification, and analysis of biological data.

I am a systems and computational biologist with over 15 years of experience in developing mathematical models to study the dynamic behavior of complex biological systems. My specialization lies in investigating the molecular mechanisms governing cell growth, division, and cell death. In addition to my research, I am actively involved in teaching Integrated Science and Systems Biology courses across all levels. Through these courses, students engage in interdisciplinary challenges through problem-oriented exercises, fostering creativity and analytical thinking. My expertise and professional aspirations closely align with the VT-CMB mission to advance the quantitative understanding of biological systems and foster the growth of interdisciplinary scientists for the future.

The research interests of my lab are focused on studying breast cancer cell cycle, signaling, metastatic processes, biomarker/drug-target discovery, and the development of microfluidic and mass spectrometry technologies for the interrogation of biological systems. The proteomic technologies that we develop enable the investigation of pathways that facilitate cancer cells to bypass tightly regulated molecular checkpoints, proliferate in an unrestrained manner, metastasize, and hijack normal biological function. To better interpret the large datasets that we generate, we are further interested in developing network-based mathematical models that will help us identify novel disease genes and drug targets. The topics that we address and the interdisciplinary methodologies that we utilize for investigating biological problems enable students to evolve in a stimulating environment and interact synergistically which other to explore fundamental biological and biomedical science questions. This approach aligns well with the mission and goals of the VT-CMB Center.

My research is focused on developing mathematical, statistical, and computational models to study the epidemiological, meteorological, ecological, physiological, evolutionary, and anthropogenic processes underlying infectious disease emergence, spread, and control. Although I’m interested in infectious diseases in general, my recent work is primarily focused on mosquito-borne diseases such as dengue, malaria, Zika, and chikungunya. These diseases are emerging or re-emerging in previously naïve populations, and outbreaks are increasing in magnitude in many endemic regions. Chief among the important drivers of these changes in disease dynamics are climate change, urbanization, and global travel. My group works closely with laboratory and field researchers to build statistical models to investigate relationships between potential drivers of infectious disease and incidence, integrate empirical and observational data into mathematical and computational models to study the role of these drivers in emergence and outbreaks, and improve models of mosquito population and infectious disease dynamics to enhance our ability to predict future outbreaks. Additionally, we develop mathematical models to investigate the efficacy of strategies aimed at reducing and eliminating mosquito-borne diseases with the goal of optimizing mitigation measures to provide sustainable, equitable, and efficient approaches to reducing the global disease burden.
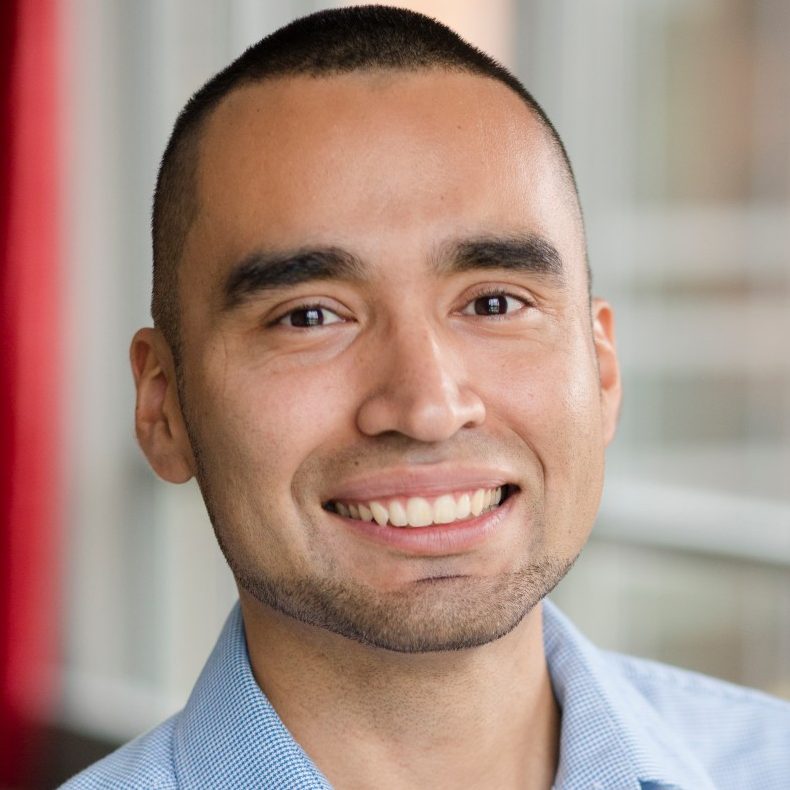
My primary area of research is in the field of mathematical epidemiology. A common theme in the work that I engage in is the union of mathematical concepts with infectious disease dynamics. I am interested in understanding how pathogens propagate through various populations, and what control policies can be implemented to mitigate or eliminate an infectious disease outbreak. In particular, I utilize deterministic and stochastic systems together with key biological components to construct and analyze infectious disease models that help address important epidemiological questions. The research projects that I have been involved in range from exploring the role of superspreaders in a population to investigating the identifiability of an SEIR model. The nature of my research is conducive to collaborations across multiple fields within the College of Science and outside of Virginia Tech, as evident through my past work. Several of my research interests, including mentoring and training of undergraduate/graduate students and postdocs, align well with the mission of VT-CMB.
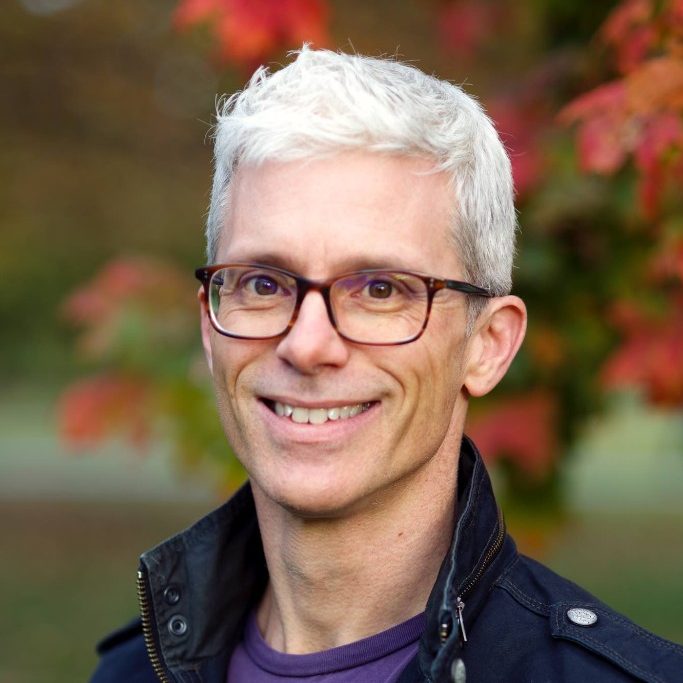
Our lab studies the biomechanics of motion in animals, conducting integrative research that crosses traditional boundaries of engineering and biology. We study how form relates to function in a diverse range of animals including snakes, lizards, frogs, birds, and insects, examining how animals move in flows and how flows are created within animals. We aim to understand the biomechanics of animals both for fundamental understanding of physiology, ecology and evolution, and as inspiration for novel engineering applications (‘bio-inspired engineering'). Our studies are intrinsically interdisciplinary, involving biology, engineering, physics, and mathematics.

My research primarily focuses on using deterministic and stochastic modeling to study the dynamics of the Spindle Assembly Checkpoint (SAC), a crucial cell cycle checkpoint. The SAC is a cellular surveillance mechanism that ensures all chromosomes are properly attached before the separation of sister chromatids during mitosis. It is both sensitive to small changes in chromosome attachment and robust against biological noise in the cellular environment. I collaborate with experimentalist to understand how the SAC achieves these seemingly opposing properties. Additionally, since last year, I have been investigating how cancer cells bypass this checkpoint, allowing them to continue growing and dividing. My interdisciplinary research aligns with the mission of VT-CMB, which aims to unite mathematicians and biologists to enhance the quantitative understanding of biological processes and human health. It also supports the goal of training the next generation of interdisciplinary scientists.

Dr. Castellano’s research is on the analysis of mathematical models for disease dynamics. In particular, he is interested in the role of spatial heterogeneity in both epidemiological models and in-host viral dynamic models. In either case, he looks for optimal strategies that allow for control over the disease. His research utilizes standard techniques from both ordinary and partial differential equations.

Kelly Cobourn
Associate Professor of Forest Resources and Environmental Conservation
As a natural resource economist, I draw heavily on mathematics to examine human behavior in biophysical systems that evolve over time and across space. For example, my work examines the control of invasive species that spread across property boundaries, the extraction of groundwater resources, and the potential for ecosystem collapse resulting from deforestation in the Brazilian Amazon. Accounting for spatiotemporal dynamics in these systems is essential as the decisions made by individuals and governments today have implications for the long-run sustainability of ecosystems and societal well-being.
I most often draw on optimal control theory and dynamic programming to develop bioeconomic simulation models that integrate economic decision-making models with dynamic constraints characterizing the ecosystem upon which people depend (e.g. insect population growth models or the movement of groundwater). To do so, I have collaborated extensively with scientists from other disciplines, such as biology, entomology, hydrology, and computer science. I am interested in developing new collaborations with mathematicians and modelers of biological processes to explore new approaches and tools that can be used to advance the conservation of natural systems.
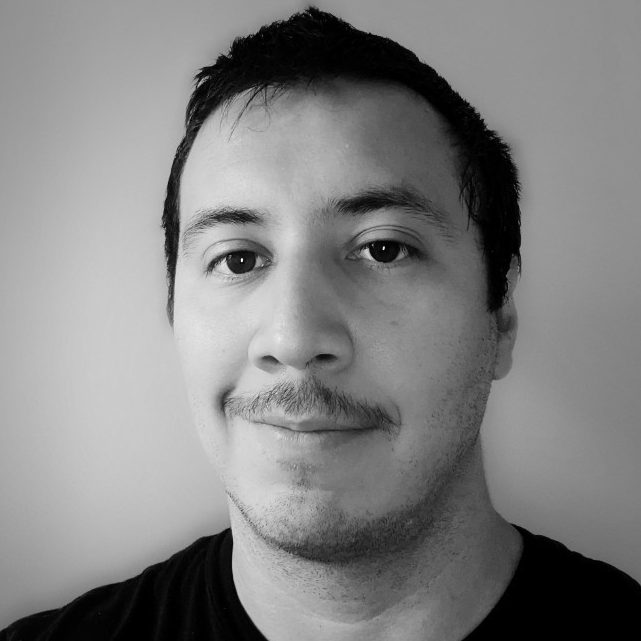
My research focuses on the cellular and molecular basis of organismal behavior within a large heterotrich ciliate genus known as Stentor. Because Stentor are unicellular organisms, their behavior can be evaluated from the perspective of signaling and cytoskeletal proteins. My research will combine cell and molecular biology with computational models of cytoskeletal and behavioral processes as well as computational/statistical models of decision-making and learning.

My research integrates advanced mathematical modeling with evolutionary ecology theory to address critical challenges in cancer treatment. I focus on three primary approaches: 1) employing optimal control techniques to identify the best treatment strategies based on the complex evolutionary dynamics of cancer cells, 2) applying spatial statistics to analyze spatial patterns and structures, thereby understanding the distribution, interactions, and dynamics that define the ecological and evolutionary niches of cancer cells, and 3) conducting model identification using sparse identification of nonlinear dynamics (SINDy), which allows biological data to inform the selection of the most suitable mathematical models. These methods together can refine clinical treatment strategies, improving the quantity and quality of life for patients through more personalized therapeutic approaches. My work extends beyond traditional academic boundaries, engaging with a diverse array of researchers and clinicians, ensuring that the innovative models and strategies developed are effectively translated into clinical solutions. This not only advances our understanding of the underlying biology of cancer but also contributes to training the next generation of interdisciplinary scientists, aligning with VT-CMB’s mission to foster collaborations and advance the quantitative understanding of biological systems.

Dr. Dahlin's research integrates ecological theory and mathematical tools to improve our understanding of the transmission of mosquito-borne parasites. His research utilizes a diverse array of mathematical modeling techniques such as ordinary differential equations, stochastic differential equations, individual-based models, and optimal control theory.
His recent work focuses on using mathematical models to identify the traits that make certain animal populations more likely to cause pathogen spillover -- the spread of diseases to human populations.

Solving large-scale optimization problems, nonlinear (and linear) systems of equations, inverse problems/parameter estimation, model reduction, stochastic methods, and application of these methods in science, engineering, and data science. These methods are important for a wide range of applications, including biology, and are areas of growing interest in the life sciences.
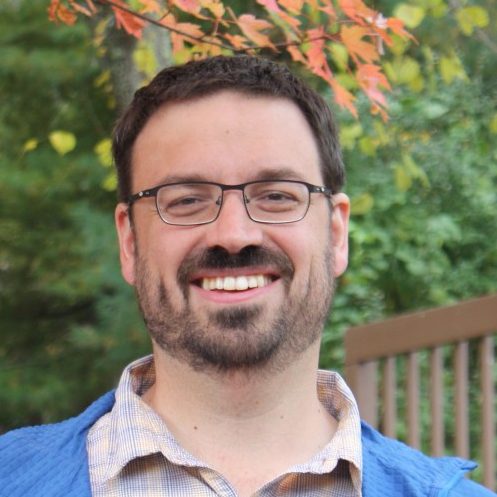
My research uses computational models of organisms and populations as my primary tool for investigating questions in evolutionary biology. Much of this work looks at large, agent-based models evolving in dynamic, heterogeneous environments over thousands of generations. Examples include models examining the fundamental evolutionary relationship between robustness and evolvability (Draghi, Parsons, Wagner, Plotkin 2010 Nature) and the evolutionary and ecological responses to environmental change in threatened populations (Draghi, McGlothlin, Kindsvater 2024 Proc B) Other projects have featured much more detailed models of processes like gene regulation. For example, I have modeled poliovirus replication to better understand its mutational process (Schulte, Draghi, Plotkin, Andino 2015 eLife) and stochasticity in gene expression to understand how cells can evolve more robust and consistent phenotypes (Draghi and Whitlock 2015 Evolution). Recent papers by my student advisees continue to apply these methods (Miller and Draghi 2024 Evolution Letters). In short, computational modeling has always been central to my work, and I believe my experience applying these tools at different scales, and to various problems, can benefit VT-CMB.Affiliated Member, Core Faculty
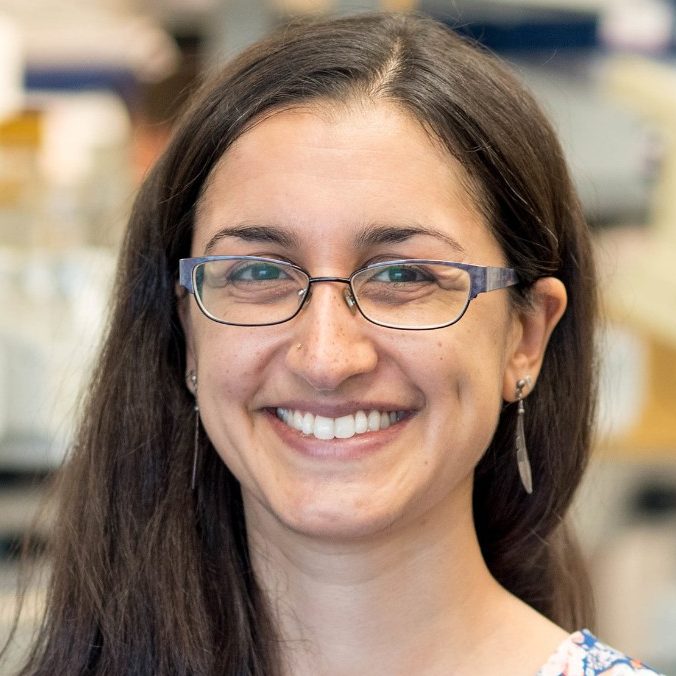
My research focuses on the transmission and pathogenesis of emerging viruses including flaviviruses and coronaviruses. We use animal models to study mosquito-borne transmission of West Nile virus and closely related viruses, sexual transmission of Zika virus, and airborne transmission of SARS-CoV-2. We are interested in the mechanisms of virus dissemination within the body and between individuals.

I am an infectious disease ecology who primarily studies the ecology and evolution of pathogens of wild bird populations. Current work focuses on how prior exposure to pathogens (and the incomplete immune memory that it generates) generates population-level heterogeneity in susceptibility, and the epidemiological and evolutionary consequences of this heterogeneity. I am an experimentalist who collaborates extensively with mathematicians and quantitative biologists to both build theoretical models that can generate testable predictions, and to parameterize models with empirical data. Although my work is primarily in wild bird populations, the questions that my laboratory asks are basic questions that are often directly applicable to human systems, which is why our heterogeneity work is currently funded by NIGMS.

I am an applied statistician working at the intersection of ecology, mathematics, and statistics. More specifically, I explore mechanistic modeling approaches to understanding how temperature impacts VBD transmission and Bayesian methods for ecological modeling.
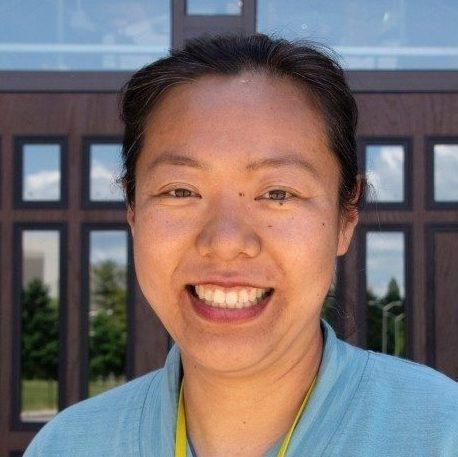
We study circadian rhythms. We are interested in understanding 1) how the circadian clock regulates the rhythms of thousands of mRNAs and proteins with the correct period, phase, and amplitude; and 2) how circadian clock utilizes rhythmically expressed proteins to regulate rhythmic physiology and behavior. We use the mouse as an animal model system and integrate diverse approaches – genetics, genomics, bioinformatics, neuroscience, molecular/cellular biology, and mathematical modeling – to answer these questions.

My main research interest centers around mathematical modeling using ordinary differential equations and dynamical systems as well as difference equations. Particularly, I am interested in modeling biological systems, primarily in epidemiology and ecology. My recent work focuses on understanding the role of human behavior in epidemic models; I have also completed projects focusing on the formulation and analysis of population models in infectious diseases to study the evolution of pathogen-host systems. I use standard deterministic methods integrated with persistence theory, stochastic processes, and programming methods using MATLAB. My goal in research is to build models to increase understanding of disease dynamics which in turn informs optimal strategies for controlling outbreaks, ultimately seeking to improve the human condition. With focuses on population dynamics and epidemiology, my research spans many different fields within the College of Sciences; collaborations with scientists across a variety of fields, within and outside of the university, are thus crucial for development of my research.
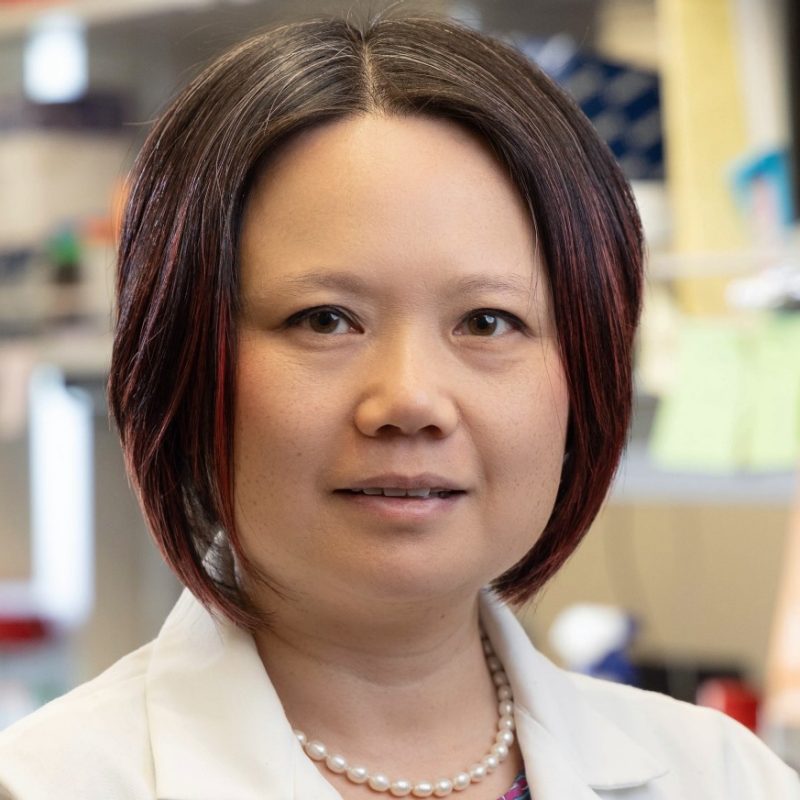
I am an immunologist and my research interests are autoimmunity, host-microbe interactions, neonatal immunity, and nutritional immunology. I have been collaborating with Dr. Stanca Ciupe on mathematical modeling of immune functions.
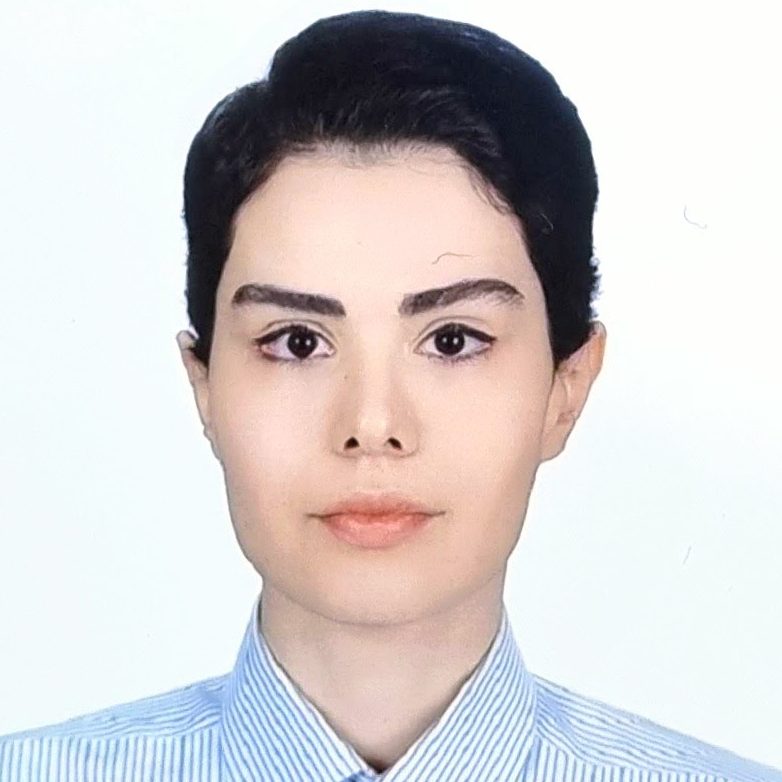
I am working on optimization of epidemic supply chains with equitable access. I use mechanistic epidemic models and the theory of optimal control to tackle infectious diseases under limited resources. I incorporate behavioral factors into the spread of the diseases and want to provide an integrated framework to take account for the dynamics of the diseases as well as resource and behavioral constraints. Unlike most research in this area, I use mathematical optimization and integer programming rather than simulation which can provide a closed form function and solid solutions without leaning on assumptions of simulation processes. This can be especially useful in unprecedented events where the insufficient information makes modeling the diseases difficult; in this case optimization techniques coupled by machine learning algorithms will provide a useful solution. After offering such framework, I compare the performance of classical simulation techniques with the suggested approach.

My research focuses on modeling the mechanics and dynamics of soft, slender structures in both natural and engineering contexts. A particular focus is on how biological creatures control slender structures. Examples include snake bodies, elephant trunks, and octopus arms. By combining biological experiments with mathematical modeling, this work seeks to provide fundamental insights into how animals establish the control of these complex, infinite degree of freedom systems.
Much of my work explores concepts of ‘mechanical intelligence.’ That is, how does the mechanical interactions of these structures with their surrounding environment offload some of the taxing control computation to the physics of the compliant interaction. I am working on analyzing these slender structures through the lens of Cosserat rods, which are slender, 1D mathematical structures that can undergo multiple modes of deformation. Recently, I have also worked with collaborators to analyze octopus arm dynamics using tools from knot theory, by considering how the octopus interconverts the link, writhe, and twist of its arm in the hopes of reducing the complexity of analyzing the arm kinematics.

My research brings together mathematicians, biologists, data scientists, and experimentalists from across the university and beyond to tackle challenges in areas such as oceanic and atmospheric dispersal of microorganisms, comparative biomechanics, biological locomotion, and biological invasion modeling. Throughout my career, I have been dedicated to training the next generation of interdisciplinary scientists, having founded the Biotrans IGEP program in 2010, which has since cross-trained over 25 PhD students at the engineering-biology interface, and more recently, training over 60 undergraduate researchers via an NSF Data Science Corps focused on data and decisions research at the engineering-biology interface. By integrating data-driven modeling, simulation, and experimental research, my work pushes the boundaries of dynamical systems methods and fluid mechanics to address real-world problems, including environmental transport and biological spread. This research has far-reaching impacts, contributing to improved understanding of biological systems with potential applications in agriculture, environmental safety, and human health.

I use mathematical models to understand the spread of infectious disease. Typically, I work on modeling the spread of disease across landscapes, and often work with data from mobile phones to inform population movement patterns. I've applied these models in a variety of settings, including to explore malaria elimination in southern Africa, the Greater Mekong Subregion, and Mesoamerica, and to simulate the spread of COVID-19 during the early stages of the pandemic. My work is strongly interdisciplinary, using mathematical tools to simulate the spread of disease, statistical tools to understand population movement over space and time, and big data to parameterize mobility.

My research focuses on the design of combinatorial and statistical algorithms to analyze and interpret sequencing data. Recent areas of emphasis include infectious disease evolution and transmission, cancer genome evolution, and cell fate mapping of developmental systems. I have developed novel models of cancer evolution, lineage tracing and infectious disease transmission that incorporate previously neglected, but essential, biological processes leading to important insights. I have also developed efficient algorithms that scale to the large datasets generated by rapidly advancing single-cell sequencing technologies.
My research goal seeks to uncover the fundamental mechanisms through which interactions among chromosomes in the cell nucleus regulate gene expression. The function of a cell, including gene expression, is determined not only by the letters of the DNA code, but also by how the DNA (contained in the chromosomes) is packaged tightly and intricately inside the tiny volume of the cell nucleus. This complex nuclear architecture - organization of the genome in 3D - has a profound effect on how the genes work and how the cell functions. However, the effects of chromosome attachments to the nuclear periphery on 3D genome organization and key cell functions are largely unknown. This collaborative project with Dr. Alexey Onufriev employs a tight integration between computation and experiment: computational models can explore vast amounts of spatial chromosomal configurations and many relevant parameter values to identify a small subset of the most promising ones, which will be tested experimentally. The research establishes a set of general principles that translate the 'language' of 3D chromosome organization into the 'language' of gene expression, which if of fundamental importance to biology.

Research in the Laboratory for Fluid Dynamics in Nature (FINLAB), part of the Department of Mechanical Engineering in the College of Engineering, explores fluid dynamics in natural settings such as coral reefs and insect physiological systems. FINLAB has a rich history of studying and modeling insect hearing and respiration, using mathematical, numerical, and computational approaches. Our collaborations with biologists, data scientists, and experimentalists in the College of Engineering (Jake Socha, ME dept; synchrotron imaging of insect tracheae), College of Science (Paul Marek/VT Insect Collection; parasitoid fly specimens and imaging), and the Smithsonian Insect Collection (parasitoid fly specimens) have been instrumental in this work. We are committed to training interdisciplinary scientists and have applied insights from our insect respiration studies to develop improved microfluidic medical devices that have received provisional patent protection. Thus, FINLAB's work aligns with many of VT-CMB's goals.

Theoretical condensed matter and statistical physics, with a focus on complex non-equilibrium systems, with applications that range from materials science to ecology and epidemiology. We apply numerical tools (Langevin molecular dynamics and agent-based Monte Carlo simulations) as well as analytical methods (field theory / functional integral mappings of stochastic dynamics, mean-field theory, perturbation expansion, renormalization group) to study various model systems. In the biology realm, we have worked on biochemical ligand-receptor (re-)binding, stochastic spatially extended population dynamics, and epidemic spreading.

We study a family of cytoskeleton proteins known as the septins. These proteins assemble into two complexes: a hexamer and octamer, and these complexes anneal to form filaments. Both of these complexes co-exist in the cells of the filamentous fungus Aspergillus fumigatus, as well as human cells. In Aspergillus, these complexes are developmentally regulated; however, the regulatory mechanism is unknown. We aim to use Aspergillus as a model to understand how the formation of these complexes are regulated by the cells and how it impacts the biomechanical properties of septin filaments. We have found a residue that is phosphorylated by PKC, which is thought to be involved in regulating GTPase activity and could serve as a mechanism to control complex assembly preference. These project will combine Cell biology, Biochemistry, and mathematical modeling to be able to address our biological questions. This will fits the goals of VT-CMB as these project is highly interdisciplinary and will benefit from collaboration with mathematical modelers as we do not have that expertise in the lab. The mathematical modeling is an essential component to understand what paramenters impact the biophysical properties of the septin filaments.

My lab studies the molecular, physiological, and neural basis of mosquito behavior. We are a group of experimental biologists, relying on a collaborative, integrative, and multidisciplinary approach, at the intersection between data science, neuro-ethology, molecular biology, and chemical ecology. Our long-term goal is to identify targets to disrupt mosquito-host interactions and reduce mosquito-borne disease transmission. Our research goals align with the goals of VT-CMB by producing data that can be leveraged for the modeling of biological processes, including neuronal networks, gene expression patterns, behavioral rhythms, and population dynamics. In addition, we use data science tools (dimensionality reduction, multivariate analysis, machine learning) to analyze and model our biological data.

My research focuses on developing elite soybean varieties tailored to the mid-Atlantic region using both classical and molecular breeding methods. This work aims to create herbicide-tolerant and conventional soybean varieties that are adaptable to diverse production systems, enhance market competitiveness through value-added traits, and build a broad genetic base for improved yield, pest resistance, and stress tolerance. By integrating molecular techniques to optimize seed composition, the program seeks to improve seed quality. This research aligns seamlessly with the CMB's mission by applying quantitative methods and interdisciplinary approaches to enhance biological systems. The center’s emphasis on fostering collaboration among mathematicians, biologists, and data scientists complements our efforts to incorporate sophisticated genetic analyses and modeling into soybean breeding. Through these collaborations, the program advances the quantitative understanding of plant genetics and contributes to the broader goal of improving agricultural productivity and sustainability, ultimately benefiting the human condition through enhanced crop resilience and quality.